The following is an excerpt from Kris Shaffer’s book, Data Versus Democracy: How Big Data Algorithms Shape Opinions and Alter the Course of History. It is part of TPM Cafe, TPM’s home for opinion and news analysis.
Much of the media we engage with today is selected for us by algorithms. This is true on social platforms like Facebook, Instagram, and Twitter; on video streaming services like YouTube and Netflix; on music streaming services like Pandora and Spotify; on shopping websites like Amazon; and especially in the ads we see across the internet. It is often claimed that these algorithms are responsible for boosting one point of view while censoring another — amplifying the biases of the programmers who build these tools and influencing what we believe, what we care about, and even how we vote.
It is true that algorithms influence what media we do and don’t encounter, and that influence can be strong and covert. But the truth is neither as simple nor as sinister as the narrative of algorithmic censorship. For one thing, not all the programmers would agree on what to amplify or censor. And in reality, algorithms learn biases from all of us — including, but absolutely not limited to, the biases of their programmers — and those algorithms sometimes do surprising things with the information we “teach” them.
While the math can be complicated and off-putting, the basic principles of these content recommendation engines are fairly straightforward. Familiarity with those principles, and how they interact with basic human psychology, helps us understand how the media we consume impacts what we believe and how we interact with the world. Such an understanding is essential if we want to make good policy around elections, media, and technology.
Bias Amplifier
Figure 3-1 illustrates the feedback loop(s) by which human biases are amplified and propagated through unchecked algorithmic content delivery. (While this illustration emphasizes search engines, the basic concepts are similar for platforms like YouTube, Facebook, and Twitter.) When a user performs a search, the model takes their search terms and any metadata around the search (location, timing, etc.) as inputs, along with data about the user from their profile and activity history, and other information from the platform’s database, like content features and the profiles and preferences of other similar users. Based on this data, the model delivers results—filtered and ranked content, according to predictions made about what the user is most likely to engage with.
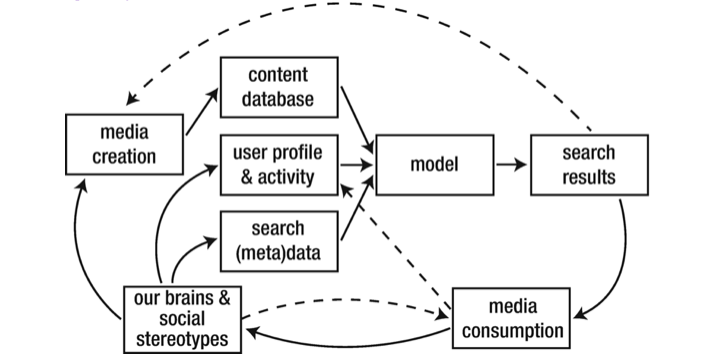
But that’s not the entire process. When we perform a search or we open up our Facebook or Twitter app, we do something with that algorithmically selected content. Let’s say I want to create a web page for a health-related event. I search for stock images of doctors and nurses to include in that brochure. When I search for an image of a doctor, the search results will be filtered according to what the search knows (and guesses) about me, what it knows (and guesses) about users assessed to have similar tastes, what content it has in its database, and what general information it knows about that content and general engagement with it. The biases about what a doctor does/should look like that are present in the world will influence the search results, and the results will in turn influence our perception of the world and our biases, which will influence further search results, etc. (Try an image search for “doctor” or “nurse” or “professor” or “teacher”. Do the search results match reality, or are they closer to common stereotypes?)
But we can add some nuance to that understanding. First, recommendation engines tend to use a technique called collaborative filtering. Collaborative filtering provides a way to fill in the gaps of a user’s profile by comparing them with other users. The theory behind it is this: if user A and user B have similar tastes for the features they both have data on, they are likely to have similar tastes for the features where one of them is missing data. In other words, if a friend and I both like distortion guitar, fast tempos, and dislike jazz, then my tastes about various classical music features will be used to make music recommendations for that friend, and their taste about country music will be used to inform my music recommendations. Our incomplete but overlapping profiles will “collaborate” to “filter” each other’s musical recommendations—hence the name.
Because of the processes of collaborative filtering, the biases I already experience and the biases of people already similar to me are the ones that will most strongly influence the output for my search. This is most starkly seen in Dylann Roof’s alleged search for “black on white crime.” Any objective crime statistics that might have offered at least a small check on his hateful extremism was masked by the fact that searches for the specific phrase “black on white crime” from users with similar internet usage patterns to Roof’s were likely to filter out the more objective and moderate content from his results and serve racist content instead.
Bias amplification can be even stronger on social media platforms like Facebook or Twitter. There the content of a user’s feed is already filtered by the people they are friends with and the pages they “like.” Since we are already prone to associate more with people like us in some way than those who are not, that already represents a significant potential filter bubble. When our past engagement data and the results of the collaborative filtering process are also taken into account, the content we see can be extremely narrow. Intervening by following pages and befriending people who represent a wider range of perspectives can only help so much, as it affects the first filter, but not the collaborative engagement-based filter. This is why close friends or family members who have many friends in common may still see radically different content in their feeds. And since both the networks of friends/pages/groups we have curated and the posts we “like” and otherwise engage with tend to reflect our personal biases and limits of perspective, the content we encounter on the platform will tend to reflect those biases and limited perspectives as well.
That leads to a second point: if the content served up to me by algorithmic recommendation is biased in ways that reflect how I already think about the world, I am not only more likely to engage with that bias, I am more likely to spread it. An increasing number of people are finding their news on social media platforms. But if it’s easier to find information in a one-stop shop like Facebook or Twitter, just think of how much easier it is to share information found on that platform. With just a tap or two, I can repropagate an article, photo, or video I encounter—without necessarily even reading the article or watching the entire video, if the previewed content gets me excited enough. And this is true for algorithmic feeds like Twitter and Facebook in a way that isn’t true for expertly curated content like that found in a print newspaper or a college textbook.
This sharing optimization compounds the filter bubble effect. Because it is easier to find information that reflects my existing biases and easier to share it, my contributions to others’ social feeds will reflect my biases even more than if I only shared content that I found elsewhere on the internet. And, of course, the same is true for their contributions to my feed. This creates a feedback loop of bias amplification: I see things in accordance with my bias, I share a subset of that content that is chosen in accordance with that bias, and that feeds into the biased content the people in my network consume, from which they choose a subset in accordance with their bias to share with me, and so on. Left unchecked, this feedback loop will continue to amplify the biases already present among users, and the process will accelerate the more people find their news via social media feeds and the more targeted the algorithm becomes. And given the way that phenomena like clickbait can dominate our attention, not only will the things that reflect our own bias propagate faster in an algorithmically driven content stream, but so will content engineered to manipulate our attention. Put together, clickbait that confirms our preexisting biases should propagate at disproportionally high speeds. And that, in fact, is what we’ve seen happen in critical times like the lead up to the 2016 U.S. presidential election. But, perhaps most importantly, the skewed media consumption that results will feed into our personal and social stereotypes about the world, influencing our behavior and relationships both online and in person.
Sometimes bias amplification works one-way. In cases like gender, racial, and other demographic representation, the dominant group has been dominant long enough that algorithms tend to amplify the pervasive bias in that same, singular direction. But this is not always the case. When it comes to politics where, especially in the United States, we are relatively equally divided into two groups, the amplification of bias is not one-sided, but two-sided or multisided. The result, then, is polarization.
Polarization is easy enough to grasp. It simply means the increase in ideological difference and/or animosity between two or more opposing groups. Digital polarization is in large part a result of the bias-amplification feedback loop applied to already like-minded groups. As biases get amplified within a group, it becomes more and more of a “filter bubble” or “echo chamber,” where content uncritically promotes in-group thinking and uncritically vilifies the opposition. Adding fuel to the fire, we also know that engagement increases when content is emotionally evocative—both positive and negative, and especially anger. This means not only more content supporting your view and discounting others, but more content is shared and reshared that encourages anger toward those outside the group. This makes it harder to listen to the other side even when more diverse content does make it through the filter.
Letting Your Guard Down
There’s another major problem that, left unchecked, causes algorithmically selected content to increase bias, polarization, and even the proliferation of “fake news.” Social platforms are designed for optimal engagement and primed for believability. Or, in the words of Renee DiResta, “Our political conversations are happening on an infrastructure built for viral advertising, and we are only beginning to adapt.”
There are several facets to this. First, social media encourages a relaxed posture toward information consumption and evaluation. By putting important news and policy debates alongside cat GIFs, baby pictures, commercial advertisements, and party invitations, social media puts us in a very different—and less critical—posture than a book, newspaper, or even magazine. Many users check social media when they are waiting in line at the store, riding the bus or train to work, even lying in bed. The relaxed posture can be great for social interactions, but that inhibition relaxation combined with the disorientation that comes from shifting between cute cats and neo-Nazi counterprotests can make it difficult to think critically about what we believe and what we share.
Second, social media platforms are designed to promote engagement, even to the point of addiction. The change from a star to a heart for a Twitter “favorite,” the increased emotion-based engagements on Facebook, all of these measures were taken in order to increase user engagement. And they worked. Meanwhile, former employees of Google, Twitter, and Facebook have gone public with ways the platforms have been designed to promote addictive behavior, and an increasing number of Silicon Valley tech employees have announced that they severely limit—or even prohibit—screen time for their own children. Promoting engagement, even addictive behavior, alongside relaxed posturing is mostly harmless when it comes to baby pictures and cute cat videos, but it is not a recipe for careful, critical thinking around the major issues of the day.
Adding fuel to this fire, a recent study suggests that people judge the veracity of content on social media not by the source of the content but by the credibility of the person who shared it. This means that even when we exercise critical thinking, we might be thinking critically about the wrong things. And platforms aren’t much help here. Most social platforms take steps to highlight the sharer (bold text, profile picture, placement in the upper left of the “card”) and downplay the originating source (smaller, lighter text, placement in the lower left of the card)—assuming the domain hosting the article is the primary source of the narrative, anyway. This only exacerbates the tendency to judge a message by the messenger instead of the source.
Psychologists Nicholas DiFonzo and Prashant Bordia study the spread of rumors online, and they have identified four primary factors that contribute to whether or not someone believes a new idea or claim of fact they encounter:
- The claim agrees with that person’s existing attitudes (confirmation bias).
- The claim comes from a credible source (which on social media often means the person who shared it, not the actual origin of the claim).
- The claim has been encountered repeatedly.
- The claim is not accompanied by a rebuttal.
In many ways, social media is engineered to bolster these factors, even for false narratives. We’ve already seen how engineering for engagement via collaborative filtering exacerbates the problem of confirmation bias and how on social media we often evaluate the wrong source. We’ve also discussed at length how repetition, especially when unconscious, can erode our ability to critically evaluate a false narrative. Both Twitter’s retweet feature and Facebook’s resurfacing of a story in our feeds every time it receives a new comment facilitate this. After all, we wouldn’t want to miss out on a lively discussion among our friends! And we know that rebuttals spread far more slowly than the viral lies they seek to correct. Social platforms as they exist today really are built to foster a sense of believability, regardless of what the truth really is.
Taking all these facts together, the deck is stacked against us for critical information consumption. Social platforms are designed in ways that make critical consumption hard and the sharing of unsubstantiated “truthy” claims easy. At the same time, more of us are getting more of our news from our social feeds, and we haven’t even talked about the role that social media plays in how print, radio, and television journalists find their scoops and frame their stories. Left unchecked, fake news, filter bubbles, and polarization will only get worse. And given the importance of an informed electorate to a democratic republic, the algorithmic news feed represents a real threat to democracy.
That said, platforms have made changes in both attitudes and algorithms over the past two years. They still have a ways to go, and new “hacks” to the system are emerging all the time, but it feels to those of us who research this problem like we are finally headed in the right direction. It’s time to capitalize on that and press forward as hard as we can, before new tactics and waning public pressure usher in a new era of complacency.
Kris Shaffer is a data scientist and a Senior Computational Disinformation Analyst for the information integrity company, New Knowledge, and has consulted for multiple U.S. government agencies, non-profits, and universities on matters related to digital disinformation, data ethics, and digital pedagogy. He co-authored “The Tactics and Tropes of the Internet Research Agency,” a report prepared for the United States Senate Select Committee on Intelligence about Russian interference in the 2016 U.S. presidential election.